The Future is No Code Cognitive Data Capture
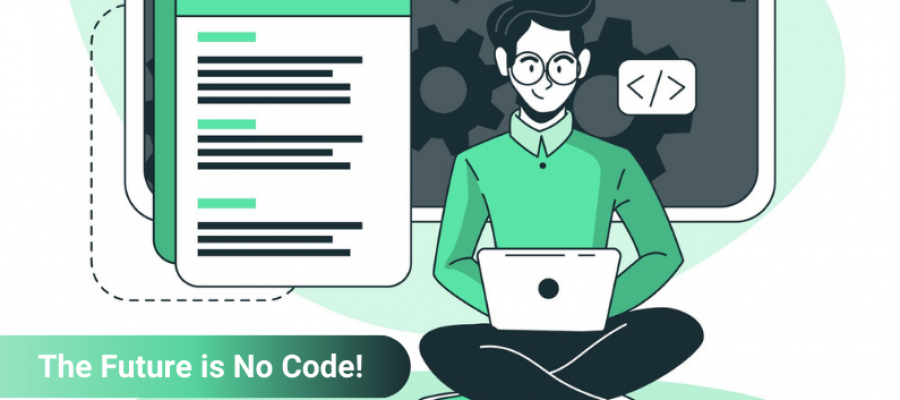
Data Capture
Data Capture
Data Capture
Data Capture
Why Data Capture and OCR were born
To understand why extracting information from documents is becoming more important, let’s do a quick recap:
The problem of manipulating data from documents is as old as the existence of electronic printers.
The invention of printers caused an explosion of printed documents sent between companies and citizens. From that point on, there have been a number of strategies deployed to meet the need of
extracting information from documents at a minimal cost.
The first strategy is just as trivial as creating checkboxes in printed documents. Checkboxes can be scanned in an efficient way using low-tech devices. However, the volume of information you can extract only using checkboxes is very limited.
The second strategy is more complex and relies on OCR (optical character recognition). OCR has been leading the race for the biggest advance in document processing for the last three decades.
However, the challenges are vast and, as the number of different document formats continues to rise, simple OCR begins to falter. In order to cope with the challenge, OCR providers started to offer document template development studios.
However, this approach implies significant cost in development consultancy to create such templates.
OCR Data Capture Limitations:
As great as OCR is, it has some important limitations. We must take into consideration that, at the end of a day, nothing less than (near) 100% accuracy is required. A bank cannot afford to wire the wrong amount of money from the wrong account, and a physician can’t get the wrong info from a clinical record.
We should acknowledge that great advances were made in OCR tech but the recognition rate still falls between 80% and 95% depending on the field. When we compute the recognition rate for a full document with 20 fields, the probability of a correct document falls well below 20%. The challenges that current OCR providers face are as follows (Please take note that this applies both to fully digital documents (PDFs) as well as photos or scanned documents):
- A high variety of document formats makes it very difficult to understand in which part of the document resides the information to be extracted
- Poorly scanned documents with low image quality
- Handwritten information that OCR cannot recognize
- Several documents mixed together on a single page
To overcome these challenges, OCR providers have been creating more and more complex platforms. With complex platforms come expensive licensing costs, consultancy and development costs, maintenance fees, and a very costly human revision operation.
As of 2021, the main avenue taken by the vast majority of companies is still this approach. An OCR project always required development services to build OCR templates. These OCR templates tell OCR software where to look in a specific document for the supplier, addresses, amounts, customer name, or any other information.
They are not building intelligence into the systems. They are just building an extensive library of cheats.
Still, OCR cannot solve its problems by itself. Companies have to deploy entire human revision operations to cleanse and correct data, which is easier now that there is an entire BPO (business process outsourcing) industry focused on data capture services.
The total cost of ownership (TCO) of an OCR project is huge (in the range of hundreds) and, for large companies, it could be in the tens of millions of dollars per year.
Digital Has Increased OCR’s Data Capture Problems
The problem continues to grow. In the last decade, every single business went digital. With digitalization and every single piece of information resting in a database, you can now virtually create any document in any format whenever you want.
Businesses are not constrained anymore by the limitations of printing, in terms of costs, operations, or mailing physical documents. With globalization, you can now adjust and create templates in real-time.
You can create templates in different languages, adapt the format by customer segment, or based on the customer’s current services portfolio. After all, this is required because humans need to understand the information that is being exchanged. Humans cannot and will not be able to process XML or JSON formats.
How big is the problem? We estimate that each company multiplied the number of templates they digitally created by a factor between 10 and 100 when compared to a decade ago.
Cognitive Data Capture or Low Code Data Capture
To tackle this problem, a new class of OCR called cognitive data capture started to arise. Cognitive data capture is nothing more than machine learning and artificial intelligence added to the old OCR toolbox.
Armed with more tools, the old OCR providers expanded their offers. Currently, almost all OCR platform solutions advertise AI and machine learning as part of their offers. Some are true but some are just marketing mimics.
The cognitive data capture solutions evolve beyond the simple rules definition of OCR templates.
By using AI and machine learning they can better recognize more generic patterns and variations, and be far more flexible than old OCR solutions. In these cognitive data capture solutions, there are a vast number of approaches, ranging from pre-training sets to real-time learning approaches with humans in the loop.
The Old OCR Data Capture Problem
The promise that the OCR problem is finally solved resembles the loss of hair tale. When you deep dive into the results, the main problem remains unsolved. Structurally, the results from pure software, either cloud or on-prem, are very far from that (near) 100% accuracy required for actual businesses.
It is true that significant advances were made in the technology and algorithms, but keep in mind that the number of document types, formats, and templates also increased exponentially.
At the end of the day, with a pure cognitive data capture tech solution, we are at the exactly same spot we were before. You need to deploy a platform, implement and configure the solution, and still run the expensive human revision operation.
Why?
Because technology alone is far from capable of extracting data from documents without errors as well as understand the subtle nuances of document structure semantics.
Because you are deploying the human operation yourself, you have neither economies of scale nor economies of experience.
A fast benchmark is comparing the TCO of the old operations and the current “intelligent” operations, taking into account its need to reach that (near) 100% accuracy threshold. The total TCO is not that different.
No Code Cognitive Data Capture
We like to think there is a better way, instead of trying to tweak and do incremental approaches that will not solve the root problem.
The root problem lies in the need for human revision as well as software, consultancy, and the implementation that goes with it.
Businesses require 100% accurate data (or very close it) extracted from their incoming documents. How you achieve that should is irrelevant. That is why we took a novel approach to the problem.
At DocDigitizer, we have a laser focus on delivering (near) 100% accurate data and, at the same time, altogether eliminating the need for human revision operations within the customer’s organization.
We still do have a human-in-the-loop. By de-constructing the all-human revision process and rebuilding the approach from scratch, we are capable of increasing productivity by a factor of five.
We christen our solution No Code Cognitive Data Capture for two main reasons:
- (near) 100% accurate data extraction. Not 80%, not 90%, not 98%, or 99%. You receive (near) 100% accurate data.
- No Code. We just need to connect to your APIs. You send documents and receive (near) 100% accurate data. It’s that simple.
DocDigitizer is leading the No Code Cognitive Data Capture revolution. The impacts for organizations are profound.
Digital transformation projects should not be blocked anymore because of a lack of data. The business case and ROI of those digital transformation projects are now easily verifiable.
DocDigitizer customers usually range from very large banks to corporations. Precisely because of our No Code approach, the medium to small-sized organizations can now also tap into the numerous benefits of end-to-end digital transformation initiatives.