The Dark Side of Machine Learning OCRs in Data Protection
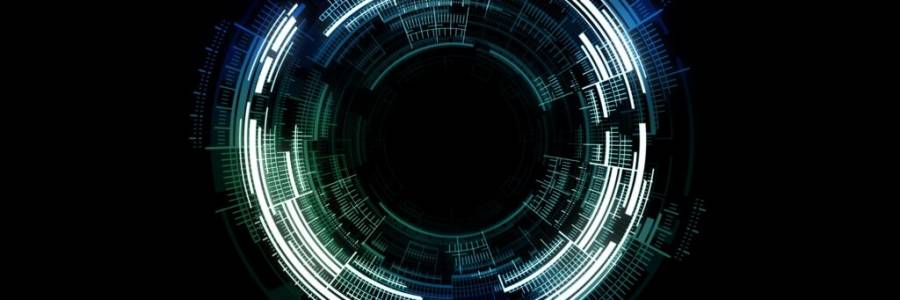
The Dark Side of Machine Learning OCRs in Data Protection
Does GDPR Or HIPAA Ring A Bell?
They are just two of the several Data Protection regulations that enforce specific compliance frameworks to organizations on the way they manage and protect their data.
These regulations have a significant impact on software systems since most data is currently handled in digital format.
In particular, since most sensitive data is exchange in the form of documents, these regulations strongly impact document management processes and OCR implementations.
From a simplistic perspective, both ECM (enterprise content managers) and OCR (optical character recognition) are essential tools to help organizations implement compliant processes.
These technologies help organizations understand the meaning of each document exchange, while also providing the means to store data securely and manage access to a digital archive.
The transparency and reliability provided by such systems are essential to reduce compliance risks and ensure that proper audit tools are available.
But is everything positive? No! This article discusses a significant challenge behind the use of modern OCR technologies for handling and processing data and how it may impact your Data Protection compliance.
Modern OCR Technologies or OCR 2.0
In recent years, there has been a quite significant change over the OCR landscape. More and more vendors are introducing Machine Learning and Deep Learning as core technologies in their products.
Conceptually, Machine Learning techniques will be able to change the value proposition behind standard OCR technologies significantly.
This is not only because they might improve data capture accuracy but also because they might reduce the need for complex and manual configurations that, nowadays, have a significant impact on the entry costs of these technologies.
Let’s take a step back and understand the basis of Deep Learning techniques.
Deep learning is a machine learning technique that teaches computers to do what comes naturally to humans: learn by example.
Deep learning is a machine learning technique that teaches computers to do what comes naturally to humans: learn by example.
Deep learning models are dependent on training, most of the time based on historical data (often called a training dataset).
In practice, companies may use their documents history as input for a Deep Learning model to configure their OCR data capture engine. By doing so, they may avoid the implementation of static, hard-coded rules that many times change from layout to layout (templates).
Moreover, Deep Learning models may take into consideration a significant amount of extraction variables, many of which are inferred by the model itself. These models process enormous batches of data under a few minutes and improve extraction quality over time without the need for human intervention.
If fed with the correct amount of training, deep learning models can achieve state-of-the-art accuracy, sometimes exceeding human-level performance.
Cloud Computing and Machine Learning are at the core of the next generation OCR technologies.
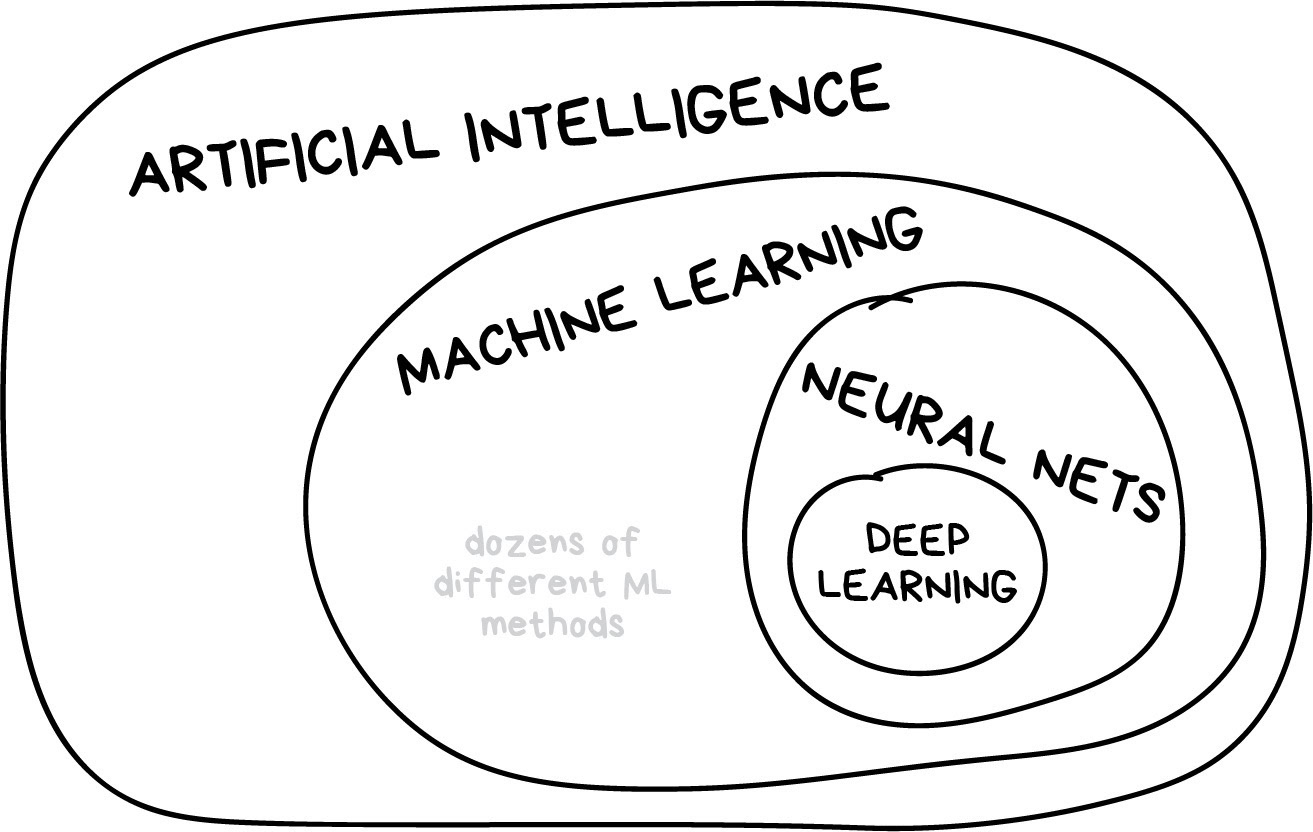
So What Is The Impact Of Machine Learning-Based OCR On Data Protection Regulation?
Most modern OCR technologies have security and privacy as a top priority. Vendors provide a wide range of security assurance policies over data protection regulations and compliance frameworks.
But if this is true for simple things such as data geolocation or document removing, researchers show limited success in getting rid of data present within Machine Learning models.
When trained with historical data, OCR and Data Capture models will infer by themselves which information is relevant and which is not, and then build an internal intricate knowledge base that cannot be easily decoded by humans.
That’s no good in an age where individuals can request their personal data to be removed from company databases under privacy measures like Europe’s GDPR policies.
So, how do you remove a person’s sensitive information from a machine learning model that has already been trained?
The reality is that it’s not possible to simply pull out discretionary parts of the knowledge base.
“Deletion is difficult because most machine learning models are complex black boxes, so it is not clear how a data point or a set of data points is really being used," as stated by James Zou, an assistant professor of biomedical data science at Stanford University.
One approach would be to retrain the model, removing the data that need to be removed. But such a solution is often not feasible, both because it requires to persist all raw information and also because it requires significant processing power, which costs time and money.
The reality is that there are no close answers to this problem. Technology is advancing at a faster pace than regulation, raising issues that are not trivial to understand or address.
Data deletion in such models is not entirely impossible, but there are still no standard tools to support it.
How To Overcome Such Limitations?
At DocDigitizer, we view these technology trends as great opportunities. Thus, we have a continuous focus on assessing their potential on the development of next-generation data capture engines.
Our proprietary technology stack leverages several of these technologies, as we are entirely confident in their potential to change the way the world exchanges and digests information.
At the same time, we make security and privacy a priority, following industry best practices to protect our customer’s data.
Balancing technology advances and compliance is at the core of our product strategy.
As a result, we have a continuous prototype pipeline, where we evaluate the potential and impact of different data capture approaches. In consequence, we collected a significant amount of lessons learned about the good, the bad, and the ugly of using Machine Learning within a Data Capture service.
One significant insight we take from this experience was the impact of model opacity not only on data deletion but also on system audits. Black box models impose severe restrictions on data management and output explicability.
Gradually we have been moving away from deploying such models within our main data flow, thus focusing on more transparent and open models.
This approach ensures that we have an end-to-end track of data and decisions made over data and that we may perform several steps of anonymization while, at the same time, not losing the ability to support continuous learning.
Tracking this data flow also allows us to easily segment training data and remove or recover critical data by request while not impacting the overall training.
We don’t see our approach as a commercial advantage. Instead, we see it as a sincere contribution to the development of a more sustainable, transparent, and secure machine learning system compliant with present and future regulation.
If you have any insight over similar or alternative strategies to deal with this challenge, please drop us a line or comment below.
Start now your digital transformation!
Related Content: WHAT IS THE RETURN ON INVESTMENT OF IMPLEMENTING OCR AUTOMATION IN MY COMPANY?