The Challenges of Traditional Optical Character Recognition
As organizations transition more and more to remote work, document processing has become one of the key functions of continuing business functions. Without a physical central office to work out of, tangible printed pieces of documents naturally become less of a boon and more of an inconvenience—as is the process of digitizing all that information. As we move into a more permanently digital age—shoved into face-first into it by the COVID-19 pandemic—transferring all those reams of paper into code has become essential, whether manually or by more advanced means, such as optical character recognition technology.
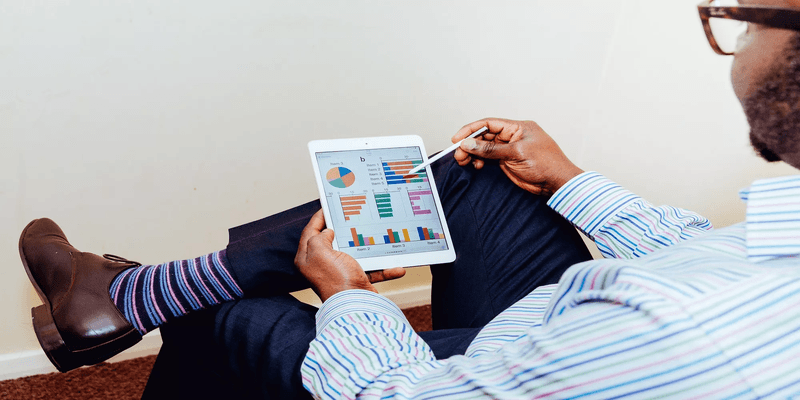
It’s an arduous task, but must get done. One doesn’t realize just how even the most basic business functions relied on physical documents until stuck in a digital work environment without them. Office communication memos, client documentation, acquisitions, accounting—all of these hinge on paper, paper, paper (or now, digital text, digital text, digital text).
In comes optical character recognition technology. Also sometimes referred to as optical character readers, or in both cases OCR for short, optical character recognition is the use of machines to convert images of documents into digital data. Rather than manually recreating the document digitally, optical character readers will scan a document and translate it into its new medium automatically. It’s a time-saver, and a lifesaver.
While the ‘wins’ of digitization are obvious—enhanced collaboration, automation adoption, saved space and time—of course difficulties and snags abound. After all, it’s human beings who have the excellent reading, writing, and thinking capabilities. Computers excel at simple analysis that doesn’t require critical thinking about what to include or leave out, what’s crucial and what’s perhaps a mistake. See why even in our burgeoning digital age data analysts still must figuratively hold computers’ hands by guiding them with exact, specific commands.
Document processing, while rote, relies on human analysis interact to ensure the final products are in fact correct. Traditional optical character readers are notoriously slow, and don’t do well with complex data. Many don’t bother converting documents into something interactive with their new interface. And even when they do, the amount of human labor required to get around these issues drains that gained time all over again. Thankfully, newer solutions are now built specifically to address these concerns in traditional OCR.
Issues With Traditional Optical Character Recognition Tools
The introduction of the first optical character reader—Edmund Fournier d’Albe’s Optophone in 1917—and subsequent innovations were a massive marker in early transitions to tech-based solutions for documentation. Of course, these solutions were and remain quite basic compared to the standards for optical character recognition demanded by the 21st century.
Innovators conceived the earliest OCRs to assist the blind and sort simple documents, like the U.S. postal service’s mail sorting process. Their optical recognition sensors could process the letters of the Latin alphabet as well as basic Roman numerals.
Unfortunately, despite these early advances optical character recognition tools more recently has had a reputation for being painfully slow and stagnant. OCR technology has barely evolved over the past decade, making devices based on its functionality run extremely slow. Think, for example, of the ubiquity of simple flatbed scanners in offices still, without any real updates made to them as tools.
The reason for this stagnation is a lack of a driving force behind the adoption of this document processing technology. Organizations that rely on OCR have found no genuine reason to change legacy systems, putting up with their many shortcomings since they find them “good enough.”
Legacy optical character recognition tools are quite resource intensive. Organizations must invest excessive human and technical resources just to make document processing viable, but they’ve done this for so long that they’ve become accompanied to the bloat and inefficiency.
OCR devices demand lots of processing speed and virtual storage daily. This usually translates to slow, weighty systems incapable of scanning large volumes of documents efficiently. In many situations, when a department needs to process several cabinets of documents, all the optical character readers are dedicated to this task, meaning no other division can access them during this period.
Legacy optical character recognition tools are also notoriously inaccurate if the document images aren’t crystal clear. Scanning low-quality documents will usually produce poor results—we’ve all experienced this frustration. But it’s unrealistic to expect that an organization will only have to process high-quality permanent media.
Organizations using OCR end up investing in teams of experts whose sole task is to check processed documents for inaccuracies and correcting them. At this point, one is processing documents twice-over—once by the machine, then again to make sure the machine didn’t mess up.
One might think it should be easy to adjust for these problems. But updating legacy optical character recognition tools is also a pain, since they are often bundled with additional e-discovery suites. The logic follows that any improvements made on one of the services must be extended to every solution within that package. But in practice, the lack of a dedicated OCR tool means one must deal with the unnecessary bloat while unable to make updates when needed.
Engine Failure to Interpret Complex Data
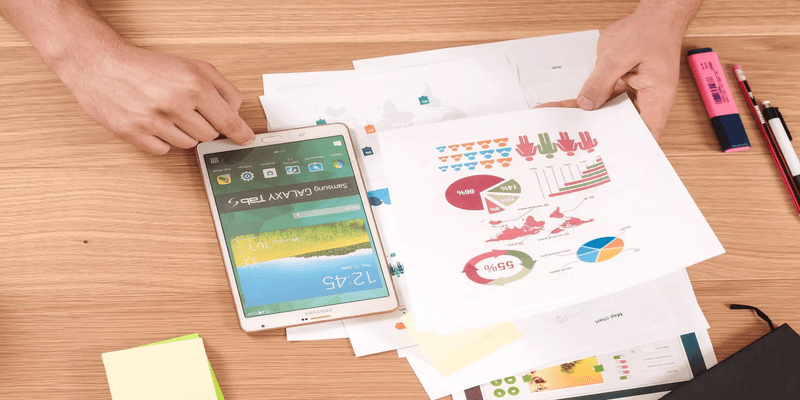
The reason traditional optical character recognition technologies often fail when presented with complex data has to do with their engines.
A first point of failure when using OCR engines strikes when tools must analyze complex forms of input. Any deviation from the preapproved inputs—for example, text written over a line—will result in a rejection or mistranslation. And not even deviation: this also happens if a block of text is just too long. Optical character recognition tools will often mistakenly skip over the section if it doesn’t immediately recognize the pattern.
Then there’s lack of engine support for different document formats. As an illustration, most optical character readers can recognize printed text and convert it into the appropriate binary data. However, they suffer with handwritten documents, which introduces a big problem when most official business reports require human signatures for verification.
As another example, modern financial analysis depends heavily on charts and tables for data organization. Unfortunately, most OCR solutions can’t process such information, since a typical table is full of lines marking columns, cells, and rows. Processed charts end up riddled with errors to then correct manually.
OCRs lack semantic awareness, and can’t process garbage values such as blank spaces. They can’t differentiate between normal text and erroneous input, instead presenting all information with the same accuracy. An erroneous misprint on a documented ends up scanned and captured by the engine as genuine data. This means that a business analyst can’t rely on optical character recognition solutions to correct documented information.
The conventional way of handling confusing data via OCR solutions has always been producing multiple outputs. This was intended to allow analysts to compare different versions produced by a computer after completing every scan. But this is wasteful, since a human analyst then spends hours or days reviewing a single scan’s results to establish the original intent.
And yet, despite all the known problems, most industries and organization carry on holding the OCR engine as the catch-all solution for data capture. This isn’t because using legacy optical character recognition tools to scan documents has become easier in recent years. One could even argue that traditional OCR functions worse now, because of the complexity and mass of documents modern businesses process. OCR often produces low-quality output when used for modern data capture needs. Rather, it’s more likely a failure of knowledge. Most businesses are just unaware that nimbler hybrid alternatives now exist.
Document processors should be capable of capturing data with a variety of complexities. They should also be able to detect errors to save an organization’s time and resources. The hours or days wasted correcting primary and secondary mistakes is better utilized handling other critical tasks that can’t be automated or computerized, such as actual decision-making.
Lack of Cross-Platform Compatibility
Even if a processor does manage to translate material without major inconveniences, processed data is only as good as the data itself. Inabilities to process output captured by third-party software, for example, or the inability to be time sensitive, throw a wrench in the process. This results in data extraction becoming a quite challenging and expensive process.
And while one might think, given the labor duplication created by faulty traditional optical character recognition tools, why not just stick to an entirely manual process, that simply isn’t feasible either. Modern organizations deal with reams customer data every day. Most of this information must be manually processed by extracting useful values that, then later converted into machine-friendly language for further analysis. These operations alone can take days or weeks of manual labor.
Businesses would spend an unrealistic amount of time to capture and process documents entirely manually—the inevitability of human corruption or fatigue makes this risky. Manual data capture methods are also prone to errors, which can lead to poor quality management and inconsistencies in output. Investors spend significant amounts of capital whenever any costly mistakes occur such as the loss of customer records. Manual processing forces organizations to invest heavily in physical data storage solutions that are prone to corruption. Such devices eat up valuable office space, an expensive commodity in metropolitan settings.
And by the time the data analysis team is done extracting and cleaning up values the information could be outdated, rendering the whole effort useless. Consider the different ways in which time spent conducting manual data entry and processing could render basic services useless. For example, identity verification to access a private facility can’t realistically be undertaken manually. Or consider anti-money laundering screening, which must be quick, efficient, and accurate for investors to consider pumping resources into institutions and organizations. Modern financial institutions perform thousands or millions of end-user verifications every minute—it simply isn’t possible to capture data and process it from all those documents manually.
Some organizations try to get around these problems by building complex custom solutions for data capture and processing. Unfortunately, such systems usually inflate the scope of a project and result in excessive costs. The solution for document processing, analysis, and automation lies elsewhere. One needs solutions that minimize both manual processing and OCR complications.
Enter DocDigitizer—The Hybrid Solution
Thankfully, more modern optical character recognition solutions exist now, specifically to combat these inefficiencies. DocDigitizer is a hybrid document processing tool that blends machine learning and human practices for no-code/RPA solutions. DocDigitizer’s compound frameworks intentionally marry the benefits of previous approaches: the interoperability of a no-code solution, scalability of RPA, speed of machine learning, and accuracy of a human touch.
Intelligent data capture means you no longer need to worry about converting low quality documents into digital sheets. DocDigitizer relies on machine “deep learning” to establish information concepts when scanning permanent media. Similar to the way humans process and retain information for future use, “deep learning” allows the machine to not only process documents, but retain information and learn from new patterns. Intelligent Document Processing allows your organization to work with both structured and unstructured data efficiently, giving you an edge over your competitors.
DocDigitizer also recognizes a variety of document formats, so you never have to worry about accepting files prepared using third-party services. The AI modules ensure that the service can accommodate formats not originally hardcoded onto the platform.
Their strategized effort offers the best of both manual and technological practices while mitigating the pitfalls of each. Nimble hybrid solutions like DocDigitizer enable your business to lead the industry in document processing.